Email Spam Filter Machine Learning: A Critical Tool for Modern Businesses
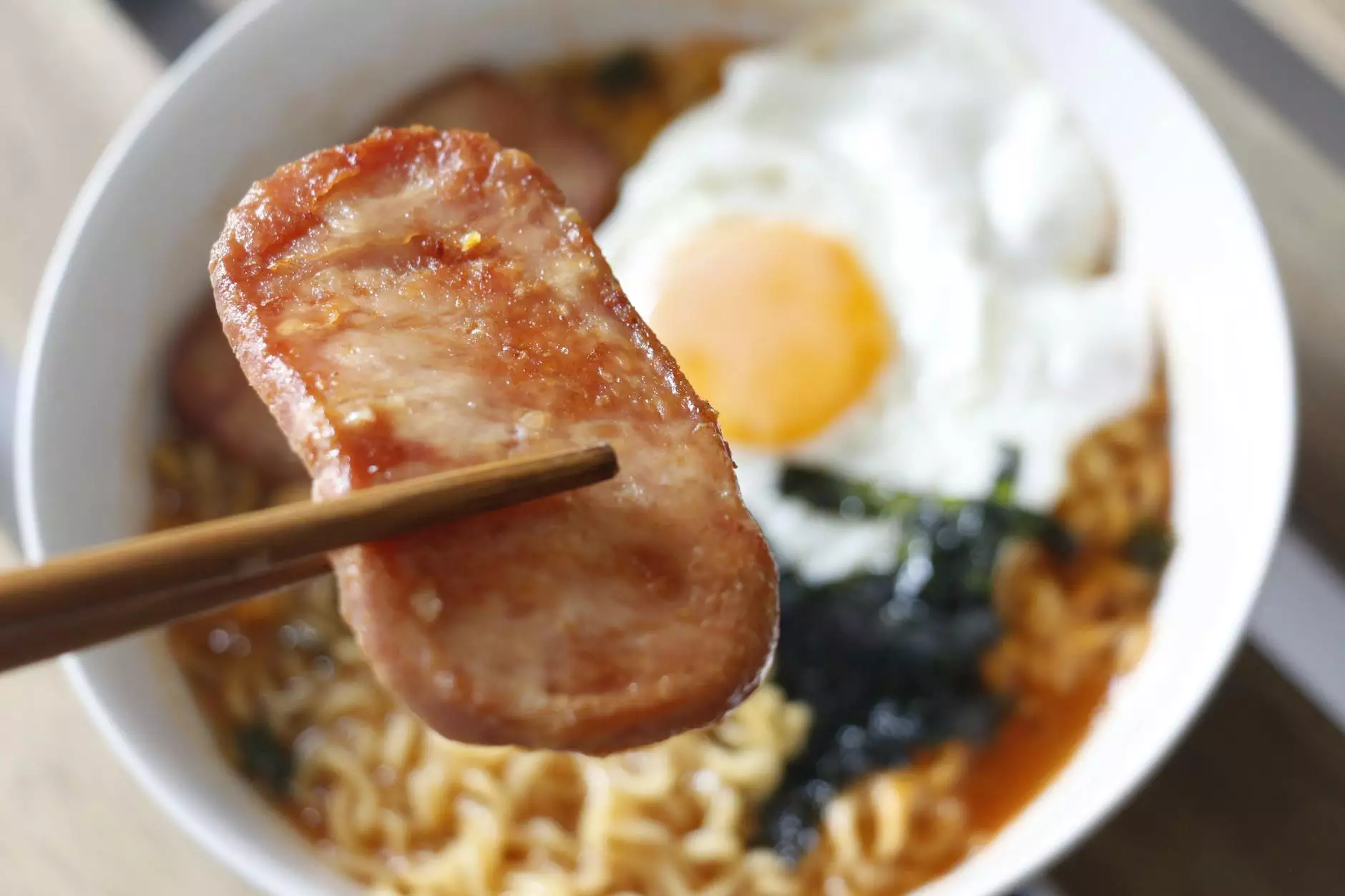
In today's digital landscape, email communication has become integral to business operations. However, the rise in email spam presents significant challenges, necessitating robust solutions to ensure that organizations remain productive and secure. One of the most effective tools in combating spam is the integration of machine learning into email spam filters. This article delves into the mechanics of machine learning spam filters, their benefits, and their significance in the realms of IT services and security systems, specifically within the context of Spambrella.
Understanding Email Spam and Its Impact on Businesses
Email spam refers to unsolicited messages sent in bulk, often with the intent to promote products, services, or schemes that may be fraudulent or harmful. Businesses face various challenges posed by email spam, including:
- Decreased Productivity: Employees spend substantial time sifting through irrelevant emails, which can detract from their capacity to focus on critical tasks.
- Security Risks: Spam emails often contain malicious links or attachments, which can lead to breaches in security and data leaks.
- Brand Reputation Damage: Sending and receiving spam can affect a company's image, as customers may associate spam-friendly policies with unprofessionalism.
- Increased IT Costs: Managing spam filters and dealing with the consequences of spam can incur additional operational costs.
The Role of Machine Learning in Email Spam Filtering
Machine learning, a branch of artificial intelligence, automates data analysis and improves the accuracy of email filtration over time. Unlike traditional spam filters, which rely on pre-existing rules and keyword matching, machine learning algorithms adapt and learn from data patterns, significantly enhancing spam detection rates. Here’s how machine learning revolutionizes email spam filtering:
1. Data-Driven Decision Making
Machine learning algorithms analyze large datasets of both spam and legitimate emails. By identifying characteristics common in spam, such as specific phrases, sender behaviors, and structural patterns, these algorithms can predict the likelihood of an email being spam with remarkable accuracy.
2. Continuous Learning and Adaptation
Machine learning models continuously evolve by incorporating new information from user interactions. When users mark emails as spam or legitimate, the model adjusts its parameters, refining its predictive capabilities. This ensures the spam filter is always up-to-date with the latest tactics used by spammers.
3. Improved Accuracy and Reduced False Positives
Using machine learning reduces the number of legitimate emails mistakenly classified as spam (false positives). As the model learns from user input, it becomes adept at discerning subtle differences between spam and legitimate communications, thereby enhancing the user experience.
Advantages of Machine Learning Email Spam Filters for Businesses
The advantages of incorporating email spam filter machine learning into an organization's IT strategy are manifold:
1. Enhanced Security
Machine learning algorithms can identify phishing attempts and malware-laden emails that traditional filters may miss. By analyzing patterns and sender reputations, businesses can mitigate risks associated with data breaches and malware infections.
2. Reduced Operational Costs
By automating the spam detection process, businesses can significantly reduce the time and resources spent on managing emails and resolving spam-related issues. This allows IT teams to focus on more strategic initiatives that contribute to the overall growth of the organization.
3. Better Email Management
Employees can experience increased productivity as they spend less time dealing with spam. Consequently, they can concentrate on tasks that drive revenue and enhance customer relationships, resulting in a more efficient workplace overall.
4. Customizable Solutions
Machine learning email spam filters can be tailored to meet specific business needs. Whether a company deals with sensitive information or requires strict compliance, machine learning can adapt accordingly, providing a personalized filtering experience.
Implementing Machine Learning Spam Filters: Best Practices
1. Choose the Right Tools
Evaluate existing email platforms and their capabilities. Opt for solutions that integrate machine learning features seamlessly while also allowing for customization based on business requirements.
2. Train the Model Effectively
Ensure that the machine learning algorithm is trained on a diverse and representative dataset. This means including a variety of spam types and legitimate emails to improve its accuracy and adaptability.
3. Monitor Performance Regularly
Regularly review the performance of the spam filter to understand its effectiveness and identify areas for improvement. Monitoring user feedback is crucial as it will guide the continued training of the model.
4. Educate Employees
While a robust spam filter is essential, educating employees on recognizing spam and phishing attempts can further enhance security. Encourage reporting of suspicious emails even if they are filtered out, and foster a culture of vigilance.
The Future of Email Spam Filtering with Machine Learning
As machine learning technologies continue to advance, we can expect even more sophisticated email spam filters in the future. Innovations are likely to include:
- Adaptive Algorithms: These will evolve continuously, picking up on emerging spam tactics in real-time.
- Enhanced User Personalization: Filters will learn individual user behaviors, thereby customizing what constitutes spam for each employee.
- Integration with Other Security Tools: Future machine learning spam filters will connect seamlessly with other cybersecurity tools, creating a unified threat detection network.
Conclusion
In conclusion, the integration of email spam filter machine learning into IT services and security systems is not just a trend but a necessity for modern businesses. With its ability to enhance security, reduce operational costs, and improve employee productivity, machine learning stands as a robust solution to the challenges posed by email spam.
Companies such as Spambrella are leading the charge in delivering innovative, effective solutions that harness the power of machine learning to create a safer and more efficient digital environment. Embracing this technology not only fortifies defenses against unwanted emails but also sets businesses on a path to sustainable growth and improved communication.